June 3, 2019
The use of artificial intelligence (AI) and algorithms is spreading rapidly across sectors. This includes areas as diverse as immigration application processing, predictive policing, and the pricing of goods and services online.
For example, decision-making algorithms have repeatedly come under fire for perpetuating existing societal biases, such as racism, and not being inclusive towards those at the margins of society, such as people facing accessibility challenges. This is particularly alarming since these critical decisions can deeply affect individuals’ lives, particularly those from marginalized groups, in detrimental ways.
Moreover, because the entire point of using an algorithm is to make a process more efficient through automation, individuals’ ability to appeal these decisions or seek redress is often limited or even non-existent. To add to this, the “black box” character of algorithmic decision-making means that many times, even their creators cannot understand how an algorithm reached its decision.
The current framework of rule instruments designed to govern the use of AI and algorithms is not up to the task of addressing the plethora of challenges that have emerged.
It is in this context that the Mowat Centre recently completed a project focused on exploring what opportunities might exist for standards-based solutions to play a larger role in governing AI and algorithms. The report produced by this research also examined two other key areas of the digital economy, namely data governance and digital platforms.
Key challenges
The use of AI and algorithms to make decisions is growing increasingly popular due to their ability to make processes faster and more efficient. Organizations are also attracted to the scalability of these automated systems.
These efficiencies, however, are not without their costs. Some of the major issues are highlighted below:
- Discrimination, bias and marginalization
AI and algorithms tend to perpetuate and even accentuate existing societal biases. This is largely a function of the fact that these algorithms are often “trained” using historical data – data which by its very nature encodes pre-existing biases. Examples of algorithmic biases are increasingly widespread. In Florida, an AI used to predict prisoners’ “risk scores” – i.e. their likelihood of committing future offences – incorrectly labelled black prisoners as future criminals at almost twice the rate of white prisoners. Other “predictive policing” systems have also been shown to further marginalize the vulnerable by disproportionately increasing police presence in low-income or non-white neighbourhoods. In Canada, a 2018 Citizen Lab report documented the use of automated decision-making in Canada’s immigration and refugee system. This report highlighted how the complex nature of many refugee and immigration claims makes this use of AI problematic, particularly because of the highly vulnerable status of the individuals involved. - Inclusion
Algorithms often disadvantage marginalized groups, such as individuals with physical disabilities, even further, due to the fact that their needs or differences are less observable or present in training data. Often, those at the edges of a normal distribution – and in many cases, at the edges of society – are marginalized in algorithmic decision-making because of how algorithms are programmed to focus on the needs of the majority who cluster at the centre of the distribution. For example, virtual assistants such as Amazon’s Alexa, can fail to understand the commands of users with speech disabilities, excluding them from taking advantage of such technologies and potentially causing distress to these users. - Transparency
A key challenge with reducing algorithmic bias, and the use of algorithms and AI in general, is their opaque “black box” nature. Even the creators of these algorithms are often unable to predict how they will respond in different situations. Additionally, algorithms are often proprietary and thus secret, meaning that accountability measures are often lacking. This is especially problematic when one realizes that, just like human decision-makers, algorithms make mistakes – often with serious consequences. Without external scrutiny, these mistakes will continue to occur for longer, and the consequences will be more pronounced. To take just one example, an algorithm used by the Government of the United Kingdom appears to have wrongly accused 7,000 international students of cheating on a test – an accusation which resulted in their deportation. - Market fairness
Another area of growing concern is the increasing use of algorithms to enable dynamic pricing. One way in which this undermines fairness is through personalized pricing, meaning that different customers are offered different prices for the same product based on their profiles, i.e. based on aspects such as postal codes or browsing history. For example, it has been found that some companies show different prices to Apple and Android users on the assumption that the former would be willing to pay more. Another common example is Uber’s “surge” pricing, a form of dynamic pricing designed to better match the supply and demand for rides through fare increases during times of high demand. Surge pricing has been heavily criticized, mainly due to its use during natural disasters and emergency situations. While Uber agreed in 2014 to cap its price increases during emergencies in the USA, the deeper question is whether a world in which different people are offered different prices by unaccountable algorithms for unknowable reasons is ethical.
Current governance landscape
The current framework of rule instruments designed to govern the use of AI and algorithms is not up to the task of addressing the plethora of challenges that have emerged.
But this does not mean that progress is not being made. For example, the European Union’s recently-adopted General Data Protection Regulation (GDPR) contains an article (22) focused specifically on algorithmic decision-making. It states that an individual “shall have the right not to be subject to a decision based solely on automated processing, including profiling, which produces legal effects concerning him or her or similarly significantly affects him or her” [Regulation (EU) 2016/679].
While this injunction suggests that the GDPR will limit the use of algorithms in making decisions that impact EU citizens, it is qualified in a number of ways elsewhere in the regulation. For example, the EU and its member states are able to make laws which allow “automated decision making” so long as they explain how these processes take “suitable measures to safeguard the data subject’s rights and freedoms and legitimate interests” [Regulation (EU) 2016/679]. Due to the newness of the GDPR, as well as the increasing novelty of ways in which AI is being used, the ultimate meaning of the GDPR’s injunction will only become clear through judicial interpretation.
In Canada, the federal government has developed a set of guiding principles for the responsible use of AI and a Directive on Automated Decision-Making which will come into full force in 2020. In terms of standards, the ISO/IEC JTC 1/SC 42 committee is a central site of standards development for AI. This committee has already published two standards on Big Data reference architecture, which are critical prerequisites for making data available and usable for the training of machine learning algorithms. Clearly, however, much work remains to be done.
What role can standards play?
Due to the global nature of Standards Developing Organizations (SDOs) and their ability to gather high-level expertise in diverse subjects to work collaboratively, these bodies are particularly well-placed to contribute to the governance of AI and algorithms. As mentioned, standards are already being created in this domain. And there is further potential for standards-based solutions.
One important area where standards can make a significant impact is on the data used to train algorithms and, in particular, through an idea called the “lawnmower of justice.” The “lawnmower of justice” is a technique designed to address the problem described earlier in which anomalous individuals are marginalized by probabilistic AI decision-making that privileges individuals with “normal” characteristics.
The technique involves “trimming” the hump in the middle of a normal distribution so that the weight of this core of concentrated data points is made less overwhelming. This rebalances the distribution so that the AI learns to pay more attention to outliers, i.e. the diverse individuals that they represent.
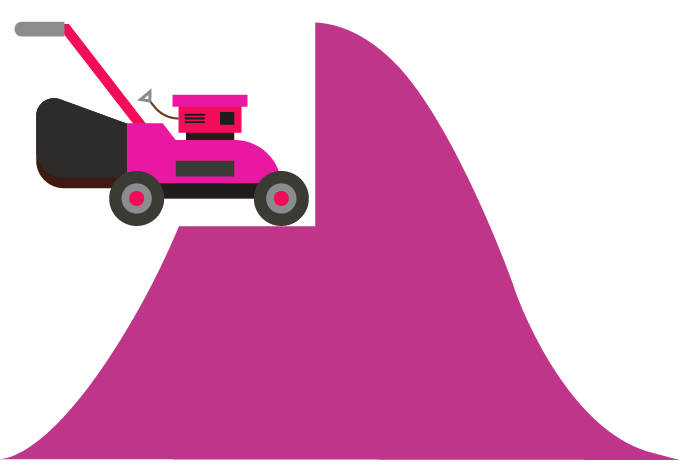
Source: Image inspired by Jutta Treviranus. Original image subject to an Attribution-NonCommercial 4.0 International (CC BY-NC 4.0) Creative Commons Licence. https://creativecommons.org/licenses/by-nc/4.0/.
Additionally, SDOs have the opportunity to develop standards for algorithmic transparency and accountability. To address unfair practices in algorithmic pricing, SDOs can work towards creating new consumer protection standards for pricing algorithms dealing with aspects such as privacy, consent and transparency.
Once these standards are established, another innovative role for SDOs could be to contribute to the creation of incubators to test these algorithms. These incubators would involve exposing algorithms to different scenarios that the algorithm might face, and then testing how it responds. Algorithms that behave or make decisions according to whatever rules have been deemed appropriate could be certified as having met a specific standard.
This TLDR is the second post in a three-part series examining the potential for standards-based solutions to play a positive role in governing the digital economy. Other posts examine opportunities for standards in the contexts of data governance and digital platforms. All three posts draw on research conducted for a project commissioned by the CSA Group which produced a report entitled: The Digital Age: Exploring the Role of Standards for Data Governance, Artificial Intelligence and Emerging Platforms.